Why addressing AI bias is mission critical for HR leaders
There’s lots of conversation about AI ethics, but how does it differ from AI bias? Harvard University’s Paola Cecchi Dimeglio shares her top tips on reducing bias from AI at work.
Expert Insight
HR leaders, as the gatekeepers of talent and culture, must take the lead on avoiding and mitigating AI biases at work.
That's the view of Harvard Faculty Chair, Paola Cecchi Dimeglio.
In an exclusive OpEd, she shares her top tips for HR leaders grappling with AI bias.
In the world of AI, bias and ethics are two distinct yet intertwined concerns that HR leaders must prioritize.
Bias in AI can lead to skewed outcomes, perpetuating unfair treatment of certain groups, while ethics encompasses the overarching principles that ensure AI is used responsibly and aligns with societal values.
For HR leaders, addressing these issues isn’t just a technical necessity—it’s a strategic imperative.
As companies increasingly integrate AI into their operations, the concern over human biases infiltrating AI systems becomes more pressing.
AI models trained on discriminatory data can scale and amplify these biases, leading to significant negative impacts.
These consequences can range from perpetuating societal inequalities to legal and reputational risks for organizations.
All of this means that addressing bias in AI is not just about fairness; it’s about achieving better outcomes, fostering trust, and ensuring an equitable workplace.
This places HR leaders right at the forefront of the fight to mitigate bias in AI for businesses.
Understanding AI bias
Let’s dial the conversation back and look at AI bias from the beginning.
AI bias, also known as machine learning or algorithmic bias, refers to AI systems that produce prejudiced results, which reflect and perpetuate societal biases. This includes both historical and current social inequalities.
Remember when Amazon discontinued its use of a hiring algorithm when it discovered that the system favored candidates who used terms like “executed” or “captured”—words that appeared more frequently on men’s resumes.
These biases can originate from the initial training data, the algorithms, or their predictions.
That’s just a brief overview; HR leaders need to understand this concept deeply if they are going to identify and address bias in AI systems effectively.
To truly understand the impact of AI bias and its ethical implications, its essential to draw from extensive experience in the field.
With over two decades of expertise in AI, big data, talent management, and leadership, I have had the privilege of advising Fortune 500 companies and government agencies on AI practices, strategic planning, decision-making, and data management both in Europe and in the US.
My work has been recognized with numerous awards from federal agencies. I have been also recognized as an expert in this field and have developed patented software and SaaS tools for unbiased performance reviews.
This comprehensive experience has given me a unique perspective on the challenges and opportunities that AI presents, particularly for global HR leaders.
Sources of and solutions to bias in AI
1. Training data bias:
-
- Definition: AI systems learn from training data, making it essential to scrutinize these datasets for biases. This type of bias occurs when the training data is not representative of the population, leading to skewed and often prejudiced outcomes.
- Example: Social bias happens when generative AI models, like those used in image generation, reflect societal prejudices embedded in their training data. For instance, these models may underrepresent women in high-performing occupations and overrepresent darker-skinned individuals in low-wage roles.
- Solution: HR leaders must ensure the use of diverse and representative AI training data by investing in high-quality data sources and employing synthetic data techniques to balance and fill gaps in datasets.
2. Algorithmic bias:
-
-
- Definition: Flawed training data can lead to biased algorithms that consistently produce unfair outcomes. This can also occur due to programming errors, where developers’ own biases influence algorithmic decisions, favoring certain demographics over others.
- Example: Recruitment algorithms favoring resumes with keywords more common among male applicants.
- Solution: HR leaders need to work closely with to ensure fair and unbiased algorithm development.
-
3. Cognitive bias:
-
-
-
- Definition: Human experiences and preferences can influence how data is selected and weighted, therefore embedding biases into AI systems. This might lead to individuals favoring data from certain regions or demographics over a more diverse global sample.
- Example: AI systems reflect the unconscious biases of their developers, such as generating images of individuals in specialized professions for job advertisements. The system depicts younger and never older individuals from Western regions.
- Solution: HR leaders should advocate for inclusive data practices that reflect a broad range of experiences and backgrounds. They should also provide fairness and data bias training for their teams.
-
-
Real-world examples of AI bias in HR
Drawing from my extensive experience working with HR leaders and businesses globally, let’s delve into some real-world examples of AI bias in HR and the solutions we can implement:
1. Recruitment, promotion and equal pay:
-
- Issue: AI-driven applicant tracking systems (ATS) have exhibited biases, favoring resumes with keywords more common among male applicants or offering certain types of feedback more often to male employees, thus reinforcing gender disparities.
- Solution: HR leaders must monitor and adjust AI tools to prevent biases and promote gender equality in hiring. Tools like Syndio, Figures or Beqom can help with pay analysis and IDEA (Intelligent Data-Driven Evaluation Analytics) or Culture Amp for continuous performance management can help identify and correct these biases.
2. Online advertising:
-
-
- Issue: Search engine algorithms have displayed gender biases, with high-paying job ads shown more frequently to men than women.
- Solution: HR leaders should collaborate with marketing teams to ensure job advertisements reach diverse audiences fairly. Tools like Textio or Applied can help avoid these biases.
-
3. Image generation:
-
-
-
- Issue: AI art generation applications have reinforced gender, racial and age biases by depicting older individuals exclusively as men in professional roles.
- Solution: HR leaders can use this insight to advocate for more balanced and inclusive representations in all company materials.
-
-
Addressing and reducing AI bias in HR
To mitigate AI biases, HR leaders must implement robust AI governance.
This means introducing policies and practices that ensure responsible AI development and use.
Some key advice from me includes:
1. Compliance:
Ensuring AI solutions adhere to industry regulations and legal standards is crucial.
HR leaders must stay informed about relevant laws and guidelines.
For instance, the US National Institute of Standards and Technology (NIST) is developing guidelines, best practices, and testing standards for the safe and ethical deployment of AI.
Similarly, the European Union’s adoption of the Artificial Intelligence Act (AI Act) on March 13, 2024, is a landmark moment for regulating AI.
2. Trust:
Building brand trust by protecting employee information and creating reliable AI systems fosters greater acceptance.
HR leaders should prioritize transparency and ethical practices.
3. Transparency:
Promoting transparency in AI algorithms provides insights into the data and processes used, ensuring fairness in outcomes.
HR leaders should demand clear documentation and regular audits of AI systems.
4. Efficiency:
Designing AI to enhance business goals, improve efficiency, and reduce costs is essential for operational success.
HR leaders should balance efficiency with fairness in AI applications.
5. Fairness:
Employing methods to assess and ensure fairness, such as counterfactual fairness, delivers equitable results regardless of sensitive attributes.
HR leaders must champion fairness and inclusion in all AI initiatives.
6. Human touch:
Integrating human oversight in AI decision-making processes maintains quality and fairness. HR leaders should ensure human review is a part of AI workflows.
7. Reinforced learning:
Using unsupervised learning techniques that transcend human biases can uncover innovative solutions.
HR leaders should encourage continuous learning and adaptation in AI systems.
This ongoing effort is crucial to staying ahead of evolving biases and ensuring AI’s long-term effectiveness and fairness in HR processes.
Moving forward on AI at work
As AI adoption grows, continuous efforts to identify and address biases will be crucial.
HR leaders, as the gatekeepers of talent and culture, must take the lead.
They need to embrace AI governance, leverage trusted AI tools, secure data, and maintain transparency to ensure AI systems benefit everyone.
By doing so, HR professionals can build AI systems that are not only efficient and innovative, but also fair and trustworthy, thereby fostering an inclusive and equitable workplace for all and driving business outcomes.
Sign up to the UNLEASH Newsletter
Get the Editor’s picks of the week delivered straight to your inbox!
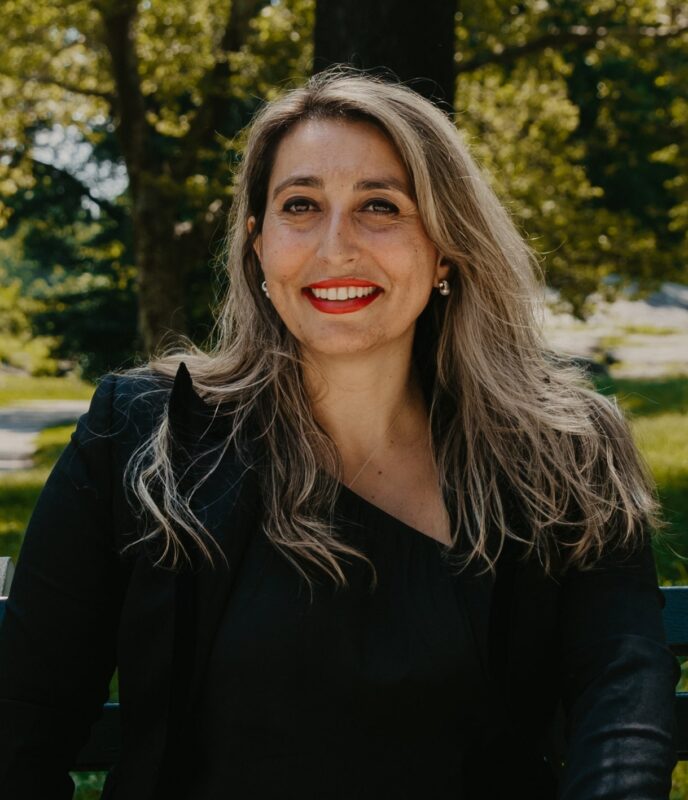
Faculty Chair
Cecchi Dimeglio is Faculty Chair ELRIWMA at Harvard University & Founder of People Culture Data Consulting Group
Contact Us
"*" indicates required fields
Partner with UNLEASH
"*" indicates required fields