A history of AI and skills with futurist David Shrier
David Shrier is a futurist who knows a thing or two about AI. Here he discusses important recent developments, as well as a look at the Bletchley Park days too.
Why You Should Care
Novelty AI or transformational AI? David Shrier knows the difference.
Discover why it's only in the last seven years or so that things have really accelerated in the AI world.
Listen above or read the full transcript beneath, which has been edited for clarity.
+++
Jon Kennard: David, thank you so much for talking to UNLEASHcast today. We’re tackling a rather large subject. It’s one that cuts across the four areas that we talk about generally, being HR tech, learning and skills, talent, and Future of Work. AI is going to play a huge part in all of those things. When we were sorting this out, one of the areas that we thought might be good to start the discussion around the digital skills gap. How can AI play a part in that one? Well, also, how can HR play a part in the closing of the digital skills gap, to you?
David Shrier: Yeah, well, I think perhaps it’s best to start with it with the second part of the question and work backwards. So, how can HR help close the digital skills gap? And the reason why I say that is because all of that is a path to an end, right? It’s a means to an end, the people who are actually driving it are in large part the learning and development organizations and the HR function within companies, because they’re tasked with stewarding the human capital of the organization.
What is important and what I think needs to assume a greater sense of urgency is the role that HR can play in bridging the digital skills gap. We are in the midst of the Fourth Industrial Revolution. We are going to see labor dislocation over the next five to 10 years. That is precedented, but it’s precedented in a scale that that’s similar to the first Industrial Revolution, where you had entire industries that became obsolete and entire new industries that were created. We need a workforce that can accommodate that.
What’s different and what HR A) needs to catch up with and B) all these technologies I was talking about can help solve, is that the pace of change is much more rapid than it ever has been before. And so we need new ways of getting effectively more knowledge into people’s heads than we used to be able to have.
JK: And so AI is the driver of this, isn’t it? You know, it’s definitely come on in leaps and bounds recently and obviously, we know that it’s a technology that’s been around an been utilized practically for decades, really. But it’s playing much, much, much more of a part now. Do you think perhaps the pandemic has changed things? Or was it a natural evolution and it was going to be becoming more popular and more widespread anyway?
DS: Yeah, so let me break that down into three parts. First of all, AI is both the problem and the solution. On one hand, AI is driving a lot of labor dislocation. It could be anywhere from 50% to, in the minds of some, more than 90% labor dislocation. It is it is changing everything and will continue to change everything. On the other hand, AI tools can help you acquire new knowledge faster and pivot into being an AI-enabled executive, so AI is both the cause and the cure, if you would. Alright, so that’s just sort of framework point one.
Point two is, yes, AI has been around for a long time. I mean, arguably, modern AI was invented by Alan Turing in the 1940s to help crack the Enigma code that was extant during World War Two. At Bletchley Park, the crypto researchers created the first AI machine. That is true.
But on the other hand, AI has only really been useful for a wide array of applications, just in the past 10 or 15 years. I mean, I wrote my first AI program in 1991. And it was sort of an interesting academic novelty, but it didn’t actually do anything terribly useful.
AI was constrained by the limits of the technology up until deep learning really came into its fore. The big breakthrough there is with another style of AI, and particularly machine learning – you can pour data into the system, and the system would get better, up to a point and then it would plateau and when you pour more data in the system, it wouldn’t get any better.
With deep learning, the more data you put in, the better it gets. This has led to a whole array of things that are noticeably improving how everything from speech recognition and your phone works to the kind of movie recommendations that Netflix makes for you. There was a change that happened only in relatively recent terms, maybe even the last seven years, around 2015, when Google started to open source their TensorFlow library that we started to see transformational AI versus narrow, specific use case AI or novelty AI.
Finally, we come to the pandemic dividend, right? I’ll call it that because the pandemic obviously caused a lot of problems, a lot of economic dislocation or disruption, but it also opened new possibilities. One of the things that it did is it showed people that remote working really could work well. People are really liking this new flexibility that is possible with digital technologies, I’m not going to restrict it to AI because there are other things that enable this remote working or digital revolution, but AI is certainly part of it.
For example, you and I are talking now, so this podcast, obviously, people can only hear my voice, but you and I are talking now, and we’re using Zoom and our backgrounds are blurred. That background blur is a machine learning driven artifact that essentially says even when you’re home, you kind of professionalize the environment a little bit. If I remove the background blur, you would see the picture of my kids on my mantel there, and some people might find that charming, but some people might find that distracting. And so, let’s start to put everybody in a neutral workspace so we can focus on each other rather than on the cool picture behind me.
AI has been around for a while, things have changed within the last seven years. And yes, COVID-19 has dramatically accelerated the adoption of digital technologies, including AI. In addition to sort of the remote workforce piece, now we can all work at a distance. And that creates all sorts of new and interesting problems for HR functions to manage when you’ve got a fully remote workforce. But also, it creates the potential for more digitization, there are a bunch of businesses that more rapidly adopted digital technology that displaced humans, and they did so during COVID because they were forced to.
JK: One thing you also actually noted…let’s go back a little bit…would it be possible for the benefit of well, not least myself, but to give me a kind of layman’s terms, potted history of deep learning? This is something that you mentioned that it sort of has changed the way that AI has been used and implemented.
DS: Yeah, so there are three major categories of artificial intelligence. First of all, let’s define what is artificial intelligence. People throw around the term, but what does it really mean? Artificial Intelligence is a machine that thinks like a human. The reason why I lean into that definition of artificial intelligence as a machine that “thinks like a human” is that we don’t know if these things are actually capable of true cognition, even now, it’s a fascinating philosophical discussion. But they do a really good job of simulating, like they are thinking like a human being or thinking at all, maybe they’re thinking in ways that are not like a human being, but they’re still thinking at a level comparable to human intelligence, if not superior to it.
Alan Turing, in his seminal paper, The Imitation Game, was positive about what is known as the Turing Test, which says, “let’s say we couldn’t see each other, we just could hear each other’s voices, or maybe we’re just typing back and forth to each other. How do you know that you’re talking to me, and not to the machine?” If you can’t tell the difference, then that machine passed the Turing Test. And it’s gotten more sophisticated over the years, but that’s the basic idea. People have elaborated the Turing Test. As AI has gotten more sophisticated, the test has gotten more sophisticated. The basic idea always was, could a machine fool a human being into thinking that they were talking to another human being?
What’s interesting is the first AI that was able to pass the Turing Test was invented in the mid-60s. Okay, so this was an Expert System, which is our first major category of AI. Expert Systems are rules-based AIs that you have to program everything that the AI does very explicitly, so there’s some human expert that’s putting their expertise into a machine.
A kind of interesting side note, the first AI to pass the Turing Test was a digital psychiatrist called Eliza. And it was specifically constructed by an MIT researcher who thought that psychiatry was all bogus, so he wanted to make fun of psychiatrists. They created this little box and people interacted with it and insisted that it was actually a human being and they were pouring their hearts out to this little engine. That engine, that Eliza, is that is the ancestor of our modern day chatbot.
Okay, so Expert Systems, first kind of AI, then we got into machine learning, which was this idea that we could create machines that had a neural network in a way. They had an engine that did not need to be explicitly programmed and could acquire knowledge by repetition of data coming into it.
That led to, for example, the original facial recognition algorithms that came about in the 80s and a number of other interesting applications of artificial intelligence. That was a first wave of innovation, and it was going along for a bit, but then it sort of plateaued. For example, I remember in the late-90s were all these speech engines like Nuance’s Dragon NaturallySpeaking. There were all these things where you could talk to the machine, and it would write down your words and it would turn speech into data. Those systems were limited because you have to train them, it was annoying to use. You had to read a very specific script that they gave you, for the machine to understand you. That’s illustrative of the overall problem of these general machine learning systems, although we still use machine learning today. Sometimes it’s quite suitable for certain applications.
Then people started trying to figure out how to stack layers of computation on top of each other in a computational model that resembled how the human brain is interconnected. I’ll use a very gross metaphor, we went from two dimensional to three dimensional. By adding that complexity to the system, the system was able to take on a lot more data, and get a lot more sophisticated.
And that’s why, starting in 2015 or so, you started to see more and more useful things happen with AI. For example, now I can talk into my phone without having to train it, I just pick it up straight out of the box, start talking to it and it will understand me pretty well, actually.
JK: I’ve got one last question. And it’s about more practical aspects in terms of skills. One thing you mentioned was how AI can help an individuals in skills acquisition, and then how HR can support that. Could you talk a little bit about that, in terms of where that’s going with skills acquisition in AI?
DS: We find that the most powerful kind of AI — when I say we, I mean not just myself but a whole group of researchers, including a number of folks at the MIT Media Lab, led by Sandy Pentland, uncovered some critical insights, among others, that you can make a very powerful system that combines humans and machines. That human machine hybrid is able to do things neither AI nor people can do alone. He’s used it to do things like predict the stock market and other future events. The other thing he’s used it for though, is to help people learn faster and better and have immediate applicability of what they’ve learned to work. What happens is, you learn with others. Collaborative learning is much more powerful, and leads to much greater retention and application than solo learning. It’s the number one failure mode of online learning, right? It’s the number one thing that massively open online courses do poorly.
JK: To be honest, we could talk maybe for two, three hours about this, couldn’t we? There’s so many applications now of AI. We’ve covered it before, and I’ve no doubt that we’ll cover it again, and the different aspects of how it can affect these four areas of content that we cover on the show. For now, David, thank you so much for talking to at UNLEASHcast today.
DS: Thank you so much. It’s been great to join you.
+++
For the full conversation – listen above…
Follow us on Spotify here
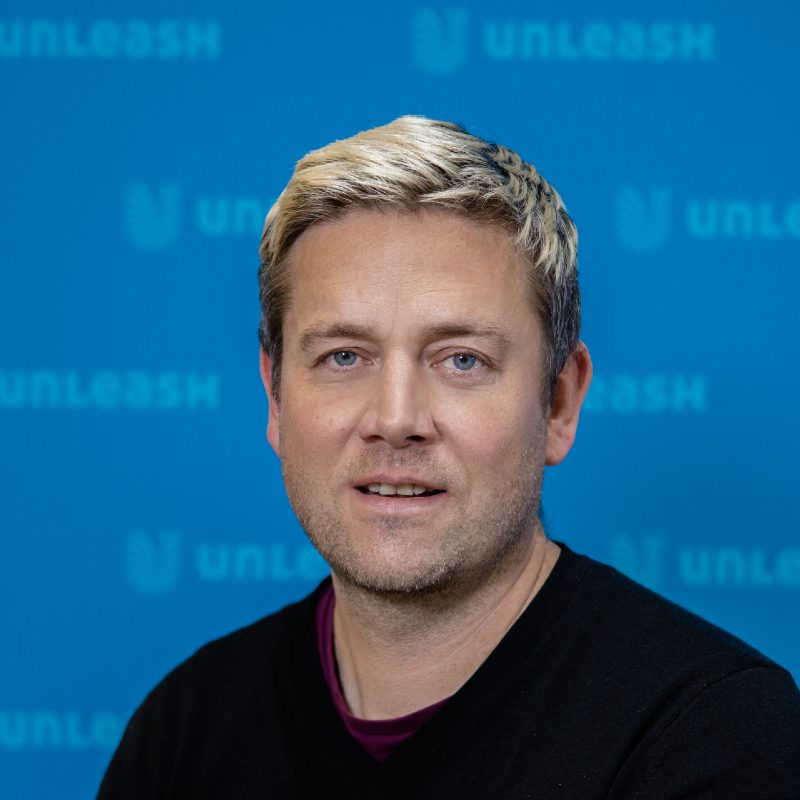
Editorial content manager
Jon has 20 years' experience in digital journalism and more than a decade in L&D and HR publishing.
Contact Us
"*" indicates required fields
Partner with UNLEASH
"*" indicates required fields